
NLP as a Golden AI Solution Reshaping Industry
Read our in depth guide all about natural language processing where we delve into the evolution of this AI discipline and how it is reshaping modern industry.
Table of contents:
From the early years of the Turing Test of 1950, there have been numerous breakthroughs and innovations within AI. Does NLP represent the current golden solution that is reshaping industries? Let’s explore the notion…
What is NLP?
Natural language processing, often referred to as NLP, is a field of artificial intelligence that focuses upon enabling computers to understand, generate and respond to human language.
Used in technologies such as chatbots, virtual assistants, sentiment analysis and translation tools, NLP bridges the gap between human communication and computer processing.
Why is it seen as so important?
NLP is heralded as a golden AI solution as it facilitates the communication between man and machine. The technology breaks down language barriers, enhances accessibility and enables intuitive responses in real-time.
It has already revolutionized many industries, and has the potential to empower many more.
AI development over time
The foundations of AI were laid in the 1950s. The Turing Test, named after prominent mathematician and computer scientist Alan Turing, was a test to distinguish between a human and a machine designed to imitate human-like responses.
Early debates surrounding AI revolved around whether to prioritise a ‘top-down’ or ‘bottoms-up’ approach. The former focused upon training the computer with the same rules that govern our human behaviour.
On the other hand, the ‘bottoms-up’ school of thought sought to create neural networks that simulated brain cells in order to learn new behaviours. Whilst both approaches have developed further, it was the top-down approach that gained the most traction initially. The US Government were early investors into AI, hoping it would provide them with a competitive advantage over the Soviet Union during the Cold War.
Later on in the 1950s, the perceptron algorithm was heralded as being “the first machine which is capable of having an original idea”. The creator of the machine, Frank Rosenblatt said:
“Stories about the creation of machines having human qualities have long been a fascinating province in the realm of science fiction. Yet we are about to witness the birth of such a machine – a machine capable of perceiving, recognizing and identifying its surroundings without any human training or control.”
Shakey the Robot was introduced in 1966, representing a breakthrough in AI and robotics. Shakey was the world’s first mobile robot that was able to think about its own actions and analyze commands, bridging the gap between logical reasoning and physical movement. The development of Shakey brought together the fields of robotics, computer vision and early natural language processing. Whilst Shakey was rather slow and cumbersome, it represented a major technological breakthrough.
The 1970s is often referred to as the AI winter. Major investment had been made into AI, with little to show for it. The British mathematician Professor Sir James Lighthill released a scathing report on the state of AI, stating that it would only ever be able to perform basic tasks at the level of an “experienced amateur” chess player. He theorized that tasks such as language processing and facial recognition would forever be beyond the capabilities of a machine.
It wasn’t until big businesses saw the potential commercial benefits of AI in the 1980s that the technology saw a renaissance. One of the key driving factors behind the revival of AI was a move away from trying to create a holistic general intelligence, and towards creating specific systems that focused on narrower tasks. An early example of a commercial AI success was the ‘RI’, developed by the Digital Equipment Corporation to configure orders for new computer systems.
1997 saw man versus machine take center stage as world chess champion Garry Kasparov took on the supercomputer Deep Blue, developed by IBM. The supercomputer was able to evaluate up to 200 million positions a second, helping it engineer a comprehensive victory over Kasparov. As noted by the BBC, some viewed this as when “AI came of age”.
The mid-2000s was defined by the successful rollout of AI-powered robots, ranging in use cases from simple home appliances such as the Roomba autonomous vacuum cleaner, right up to military equipment such as Boston Dynamic’s BigDog and iRobot’s PackBot, a bomb disposal robot that was deployed across Iraq and Afghanistan.
Eugene Goostman is a famous name in the AI world, and he doesn’t even exist! In 2014, a chatbot portraying Eugene, a 13 year old Ukrainian child from Odessa, was claimed to have ‘passed’ the Turing Test. Whilst the pass was deemed as controversial for apparently being trained to fool the judges rather than convince them, it still was a breakthrough moment for the famous Turing Test sixty four years after it was first introduced.
The 2010s can be seen as the era of deep learning, which is a field of machine learning that uses neural networks to enable machines to learn and make decisions from large datasets. AlexNet, a convolutional neural network designed by Alex Krizhevsky had 60 million parameters and 650,000 neurons. What made this model so interesting was the top-5 error rate of just 15.3%, which was seen as revolutionary, opening up new potential for the use of deep neural networks in the handling of large image datasets.
Generative AI continues to dominate the contemporary headlines regarding AI, and it is easy to understand why. Large language models such as OpenAI’s ChatGPT demonstrate unprecedented levels of natural language processing and understanding, which have helped drive widespread adoption of the tools.
According to Salesforce data, 45% of the US population used generative AI tools in 2024, as well as 29% of the British, 49% of the Australian and a staggering 73% of the Indian population.
NLP and Generative AI
Natural language processing is at the bedrock of generative AI tools such as ChatGPT and Canva’s Magic Design. Some of the uses of NLP within the generative AI solutions include:
- Helping the tools to understand the inputted queries or prompts
- Provide context to the prompt to ensure the output is contextually appropriate
- Ensure the generated text is tone-specific
- Provides multilingual capabilities
- Analyses sentiment, allowing the generative tools to respond empathetically and to understand emotional cues
- Helps the generative AI tools to extract and understand key bits of data from large datasets, so that the tools can provide factual responses
Industries being revolutionized by NLP
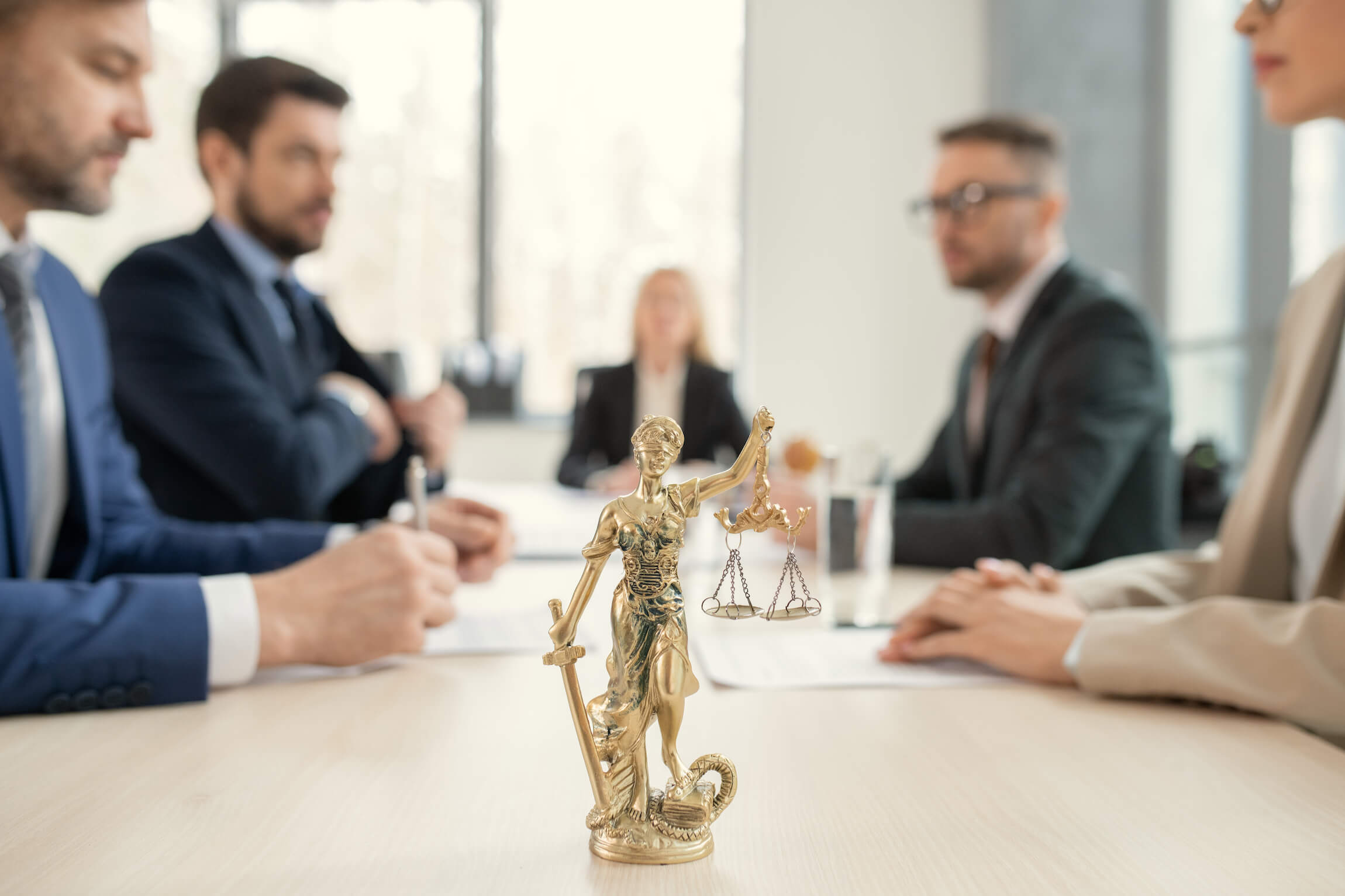
The industry possibly most revolutionized by the use of NLP solutions is the legal industry. A Goldman Sachs report in 2023 forecast that 44% of all legal tasks in the US could be automated using generative AI, something that has quickly become a reality for many legal firms. AI-powered Legal tech goes far beyond simply using ChatGPT for text summaries.
Across many prestigious law firms, these NLP-powered processes are being incorporated directly into the workflows and operations. Additionally, legal chatbots such as ‘DoNotPay’ offer a ‘robot lawyer’ to users battling parking fines. An LSE study found that as of March 2024, over 60% of large law firms were using generative AI systems.
The customer service industry has wholeheartedly embraced NLP technology. Virtual assistant chatbots powered by AI have been introduced, offering 24/7 customer support to handle common queries and provide personalized instructions.
This has proved popular for customers and businesses alike, as the wait time between the initial enquiry and the resolution has been significantly reduced. For tasks outside the capabilities of the NLP chatbot, built-in safeguards can recommend an escalation to a human customer service agent, providing the best of both worlds between automated systems and dedicated human expertise.
Healthcare is another industry that has widely incorporated NLP solutions. Apps such as the UK’s NHS healthcare app will ask pre-determined questions regarding a person’s health. In this specific instance, the app’s medical chatbot has been trained not to provide a diagnosis, but instead to signpost the user towards the most suitable provider of care.
Should the chatbot detect an urgent health issue, such as chest pains, the chatbot will automatically advise the user to seek immediate medical attention. One key consideration for the development of these medical NLP solutions is that they can only be trained on open closed, controlled medical sources as opposed to open-source information that could be of questionable medical accuracy.
You can read about how other NLP-driven methods, alongside chatbot integration, are being developed here at NetGeist to benefit the healthcare industry.
Final thoughts
NLP has firmly established itself as the golden AI solution for our age. Generative AI tools have burst onto the scene and continue to provide value for businesses and consumers alike.
Should your business be looking to integrate NLP into your workflows, NetGeist is here to help. Our custom project capabilities help businesses like yours to deliver a solution specifically designed with your customer in mind. Whether it is a chatbot virtual assistant, app review monitoring application, HR data-driven strategies or much more, we can help deliver for your business.
Chat with Assistant Robert to get started with your custom solution!